Document Type
Article
Publication Date
12-9-2021
Publication Title
Journal of Personalized Medicine
Volume
11
Issue
12
First page number:
1
Last page number:
15
Abstract
Glioblastoma is an aggressive brain tumor with a low survival rate. Understanding tumor behavior by predicting prognosis outcomes is a crucial factor in deciding a proper treatment plan. In this paper, an automatic overall survival time prediction system (OST) for glioblastoma patients is developed on the basis of radiomic features and machine learning (ML). This system is designed to predict prognosis outcomes by classifying a glioblastoma patient into one of three survival groups: short-term, mid-term, and long-term. To develop the prediction system, a medical dataset based on imaging information from magnetic resonance imaging (MRI) and non-imaging information is used. A novel radiomic feature extraction method is proposed and developed on the basis of volumetric and location information of brain tumor subregions extracted from MRI scans. This method is based on calculating the volumetric features from two brain sub-volumes obtained from the whole brain volume in MRI images using brain sectional planes (sagittal, coronal, and horizontal). Many experiments are conducted on the basis of various ML methods and combinations of feature extraction methods to develop the best OST system. In addition, the feature fusions of both radiomic and non-imaging features are examined to improve the accuracy of the prediction system. The best performance was achieved by the neural network and feature fusions.
Keywords
Accuracy; Brain tumor; Edema; Enhanced tumor; High grade glioma; Machine learning; Magnetic resonance imaging; Neural network; Tumor core
Disciplines
Computer Sciences
File Format
File Size
3150 KB
Rights
IN COPYRIGHT. For more information about this rights statement, please visit http://rightsstatements.org/vocab/InC/1.0/
Creative Commons License
This work is licensed under a Creative Commons Attribution 4.0 International License.
Repository Citation
Chato, L.,
Latifi, S.
(2021).
Machine Learning and Radiomic Features to Predict Overall Survival Time for Glioblastoma Patients.
Journal of Personalized Medicine, 11(12),
1-15.
http://dx.doi.org/10.3390/jpm11121336
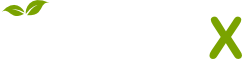
- Citations
- Citation Indexes: 15
- Usage
- Downloads: 160
- Abstract Views: 20
- Captures
- Readers: 31
- Mentions
- Blog Mentions: 1