Suspended Sediment Load Prediction of River Systems: An Artificial Neural Networks Approach
Document Type
Article
Publication Date
3-2011
Publication Title
Agricultural Water Management
Volume
98
Issue
5
First page number:
855
Last page number:
866
Abstract
Information on suspended sediment load is crucial to water management and environmental protection. Suspended sediment loads for three major rivers (Mississippi, Missouri and Rio Grande) in USA are estimated using artificial neural network (ANN) modeling approach. A multilayer perceptron (MLP) ANN with an error back propagation algorithm, using historical daily and weekly hydroclimatological data (precipitation P(t), current discharge Q(t), antecedent discharge Q(t−1), and antecedent sediment load SL(t−1)), is used to predict the suspended sediment load SL(t) at the selected monitoring stations. Performance of ANN was evaluated using different combinations of input data sets, length of record for training, and temporal resolution (daily and weekly data). Results from ANN model were compared with results from multiple linear regressions (MLR), multiple non-linear regression (MNLR) and Autoregressive integrated moving average (ARIMA) using correlation coefficient (R), mean absolute percent error (MAPE) and model efficiency (E). Comparison of training period length was also made (4, 3 and 2 years of training and 1, 2 and 3 years of testing, respectively). The model efficiency (E) and R2 values were slightly higher for the 4 years of training and 1 year of testing (4 * 1) for Mississippi River, indifferent for Missouri and slightly lower for Rio Grande River. Daily simulations using Input 1 (P(t), Q(t), Q(t−1), SL(t−1)) and three years of training and two years of testing (3 * 2) performed better (R2 and E of 0.85 and 0.72, respectively) than the simulation with two years of training and three years of testing (2 * 3) (R2 and E of 0.64 and 0.46, respectively). ANN predicted daily values using Input 1 and 3 * 2 architecture for Missouri (R2 = 0.97) and Mississippi (R2 = 0.96) were better than those of Rio Grande (R2 = 0.65). Daily predictions were better compared to weekly predictions for all three rivers due to higher correlation within daily than weekly data. ANN predictions for most simulations were superior compared to predictions using MLR, MNLR and ARIMA. The modeling approach presented in this paper can be potentially used to reduce the frequency of costly operations for sediment measurement where hydrological data is readily available.
Keywords
Artificial neural network (ANN); Autoregressive integrated moving average (ARIMA); Computer simulation; Mississippi River; Missouri River; Multiple linear regressions (MLR); Multiple non-linear regression (MNLR); North America – Rio Grande; Neural networks (Computer science); Regression analysis; Sediment prediction; Suspended sediments
Disciplines
Environmental Engineering | Environmental Monitoring | Environmental Sciences | Fresh Water Studies | Water Resource Management
Language
English
Permissions
Use Find in Your Library, contact the author, or use interlibrary loan to garner a copy of the article. Publisher copyright policy allows author to archive post-print (author’s final manuscript). When post-print is available or publisher policy changes, the article will be deposited
Publisher Citation
A.M. Melesse, S. Ahmad, M.E. McClain, X. Wang, Y.H. Lim, Suspended sediment load prediction of river systems: An artificial neural network approach, Agricultural Water Management, Volume 98, Issue 5, March 2011, Pages 855-866, ISSN 0378-3774, 10.1016/j.agwat.2010.12.012. (http://www.sciencedirect.com/science/article/pii/S0378377410003951)
Repository Citation
Melesse, A. M.,
Ahmad, S.,
McClain, M. E.,
Wang, X.,
Lim, Y. H.
(2011).
Suspended Sediment Load Prediction of River Systems: An Artificial Neural Networks Approach.
Agricultural Water Management, 98(5),
855-866.
http://dx.doi.org/10.1016/j.agwat.2010.12.013
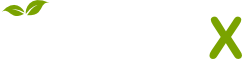
- Citations
- Citation Indexes: 49
- Policy Citations: 1
- Usage
- Abstract Views: 118
- Captures
- Readers: 69